Poetry Generation Using Tensorflow, Keras, and LSTM
What is RNN
Recurrent Neural Networks are the first of its kind State of the Art algorithms that can Memorize/remember previous inputs in memory, When a huge set of Sequential data is given to it. Recurrent Neural Networks are the first of its kind State of the Art algorithms that can Memorize/remember previous inputs in memory, When a huge set of Sequential data is given to it.
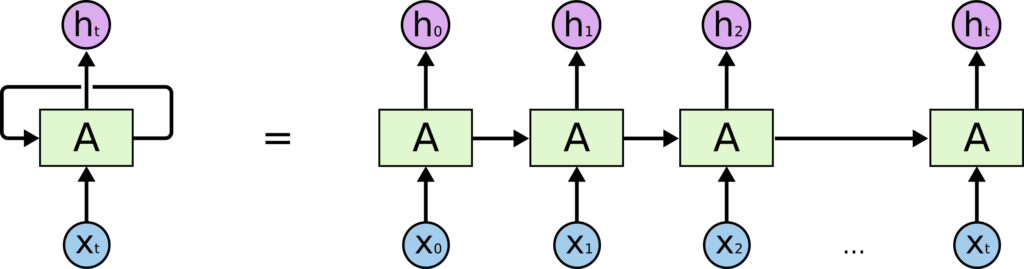
These loops make recurrent neural networks seem kind of mysterious. However, if you think a bit more, it turns out that they aren’t all that different than a normal neural network. A recurrent neural network can be thought of as multiple copies of the same network, each passing a message to a successor.
Different types of RNN’s
Different types of Recurrent Neural Networks.
- Image Classification
- Sequence output (e.g. image captioning takes an image and outputs a sentence of words).
- Sequence input (e.g. sentiment analysis where a given sentence is classified as expressing positive or negative sentiment).
- Sequence input and sequence output (e.g. Machine Translation: an RNN reads a sentence in English and then outputs a sentence in French).
- Synced sequence input and output (e.g. video classification where we wish to label each frame of the video)
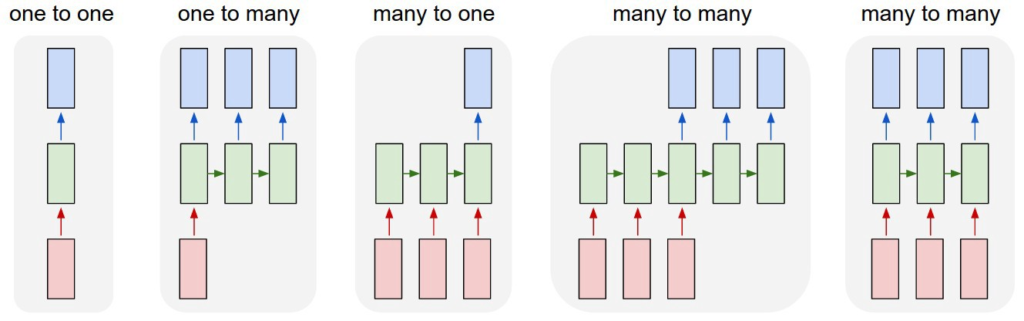
The Problem of RNN's or Long-Term Dependencies
- Vanishing Gradient
- Exploding Gradient
Vanishing Gradient
If the partial derivation of Error is less than 1, then when it get multiplied with the Learning rate which is also very less. then Multiplying learning rate with partial derivation of Error wont be a big change when compared with previous iteration.
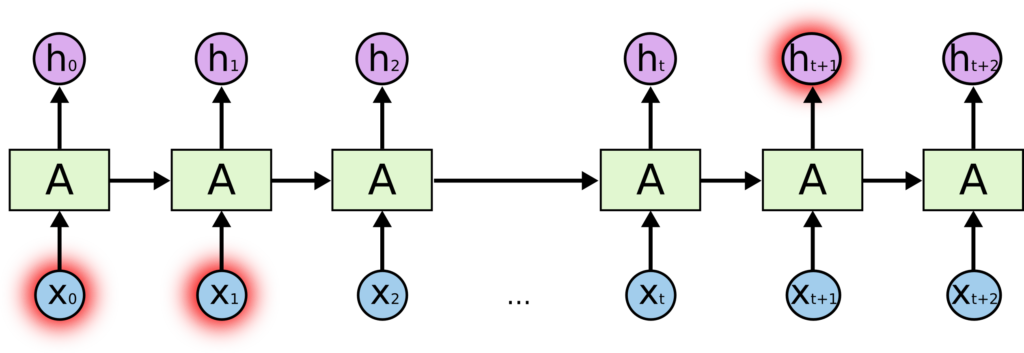
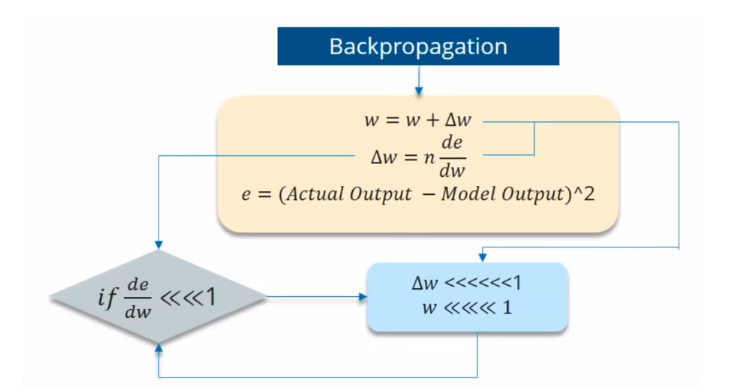
Exploding Gradient
We speak of Exploding Gradients when the algorithm assigns a stupidly high importance to the weights, without much reason. But fortunately, this problem can be easily solved if you truncate or squash the gradients
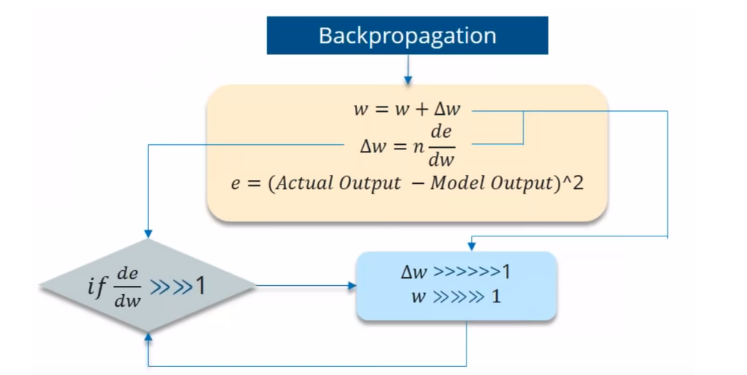
Long Short Term Memory (LSTM) Networks
Long Short Term Memory networks – usually just called “LSTMs” – are a special kind of RNN, capable of learning long-term dependencies.
LSTMs are explicitly designed to avoid the long-term dependency problem. Remembering information for long periods of time is practically their default behavior, not something they struggle to learn!
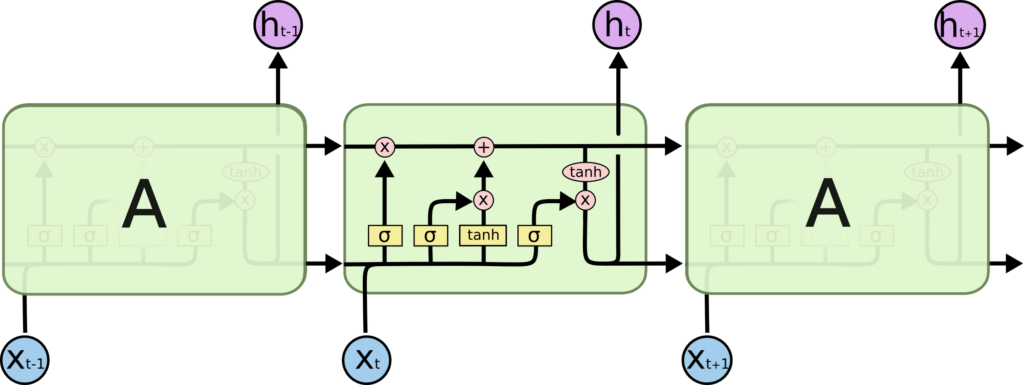

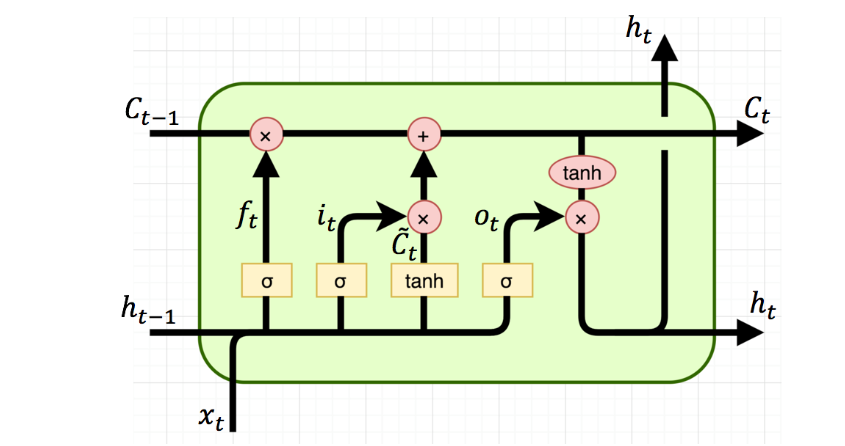
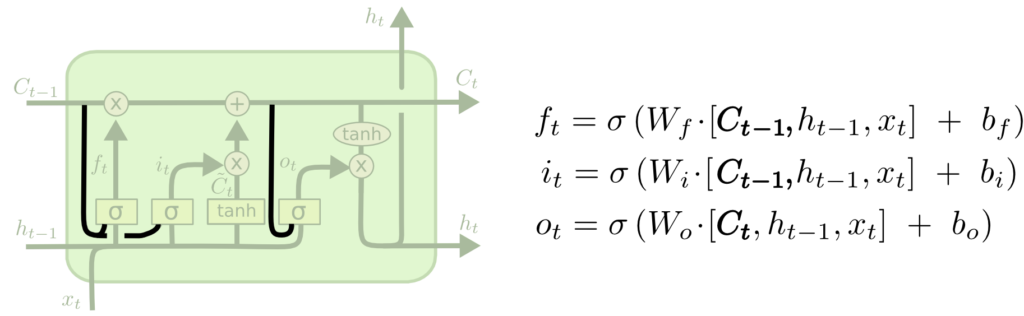
Sequence Generation Scheme
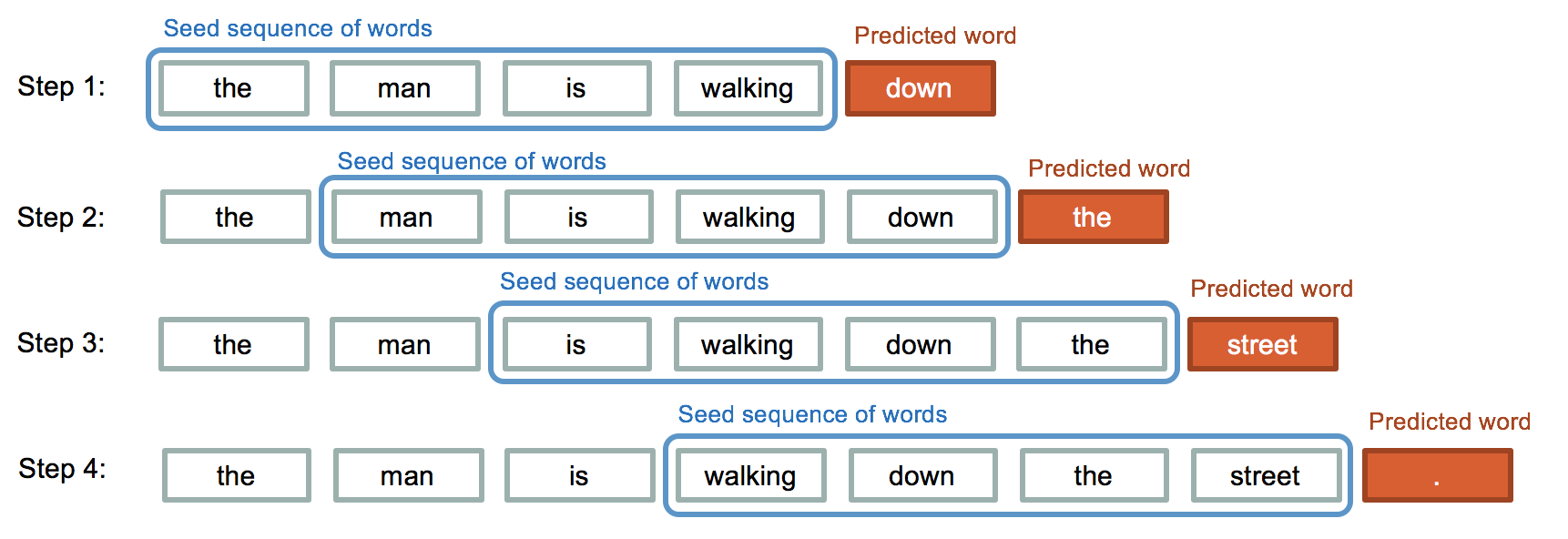
Let's Code
import tensorflow as tf import string import requests import pandas as pd
response = requests.get('https://raw.githubusercontent.com/laxmimerit/poetry-data/master/adele.txt')
response.text
'Looking for some education\nMade my way into the night\nAll that bullshit conversation\nBaby, can\'t you read the signs? I won\'t bore you with the details, baby\nI don\'t even wanna waste your time\nLet\'s just say that maybe\nYou could help me ease my mind\nI ain\'t Mr. Right But if you\'re looking for fast love\nIf that\'s love in your eyes\nIt\'s more than enough\nHad some bad love\nSo fast love is all that I\'ve got on my mind Ooh,
data = response.text.splitlines() len(data)
2400
len(" ".join(data))
91330
Build LSTM Model and Prepare X and y
import numpy as np from tensorflow.keras.preprocessing.text import Tokenizer from tensorflow.keras.utils import to_categorical from tensorflow.keras.models import Sequential from tensorflow.keras.layers import Dense, LSTM, Embedding from tensorflow.keras.preprocessing.sequence import pad_sequences
token = Tokenizer() token.fit_on_texts(data)
# token.word_counts
help(token)
token.word_index
{'i': 1, 'you': 2, 'the': 3, 'me': 4, 'to': 5, ...}
encoded_text = token.texts_to_sequences(data) encoded_text
[[254, 21, 219, 725], [117, 8, 80, 153, 3, 133], [14, 10, 726, 727], ...]
x = ['i love you'] token.texts_to_sequences(x)
[[1, 11, 2]]
vocab_size = len(token.word_counts) + 1
Prepare Training Data
datalist = [] for d in encoded_text: if len(d)>1: for i in range(2, len(d)): datalist.append(d[:i]) print(d[:i])
Padding
max_length = 20 sequences = pad_sequences(datalist, maxlen=max_length, padding='pre') sequences
array([[ 0, 0, 0, ..., 0, 254, 21], [ 0, 0, 0, ..., 254, 21, 219], [ 0, 0, 0, ..., 0, 117, 8], ..., [ 0, 0, 0, ..., 17, 198, 17], [ 0, 0, 0, ..., 198, 17, 198], [ 0, 0, 0, ..., 17, 198, 6]], dtype=int32)
X = sequences[:, :-1] y = sequences[:, -1]
y = to_categorical(y, num_classes=vocab_size) seq_length = X.shape[1]
LSTM Model Training
model = Sequential() model.add(Embedding(vocab_size, 50, input_length=seq_length)) model.add(LSTM(100, return_sequences=True)) model.add(LSTM(100)) model.add(Dense(100, activation='relu')) model.add(Dense(vocab_size, activation='softmax'))
model.summary()
Model: "sequential" _________________________________________________________________ Layer (type) Output Shape Param # ================================================================= embedding (Embedding) (None, 19, 50) 69800 _________________________________________________________________ lstm (LSTM) (None, 19, 100) 60400 _________________________________________________________________ lstm_1 (LSTM) (None, 100) 80400 _________________________________________________________________ dense (Dense) (None, 100) 10100 _________________________________________________________________ dense_1 (Dense) (None, 1396) 140996 ================================================================= Total params: 361,696 Trainable params: 361,696 Non-trainable params: 0 _________________________________________________________________
model.compile(loss='categorical_crossentropy', optimizer='adam', metrics=['accuracy'])
model.fit(X, y, batch_size=32, epochs=50)
Epoch 49/50 445/445 [==============================] - 3s 6ms/step - loss: 0.5386 - accuracy: 0.8388 Epoch 50/50 445/445 [==============================] - 3s 6ms/step - loss: 0.5385 - accuracy: 0.8371
Poetry Generation
poetry_length = 10 def generate_poetry(seed_text, n_lines): for i in range(n_lines): text = [] for _ in range(poetry_length): encoded = token.texts_to_sequences([seed_text]) encoded = pad_sequences(encoded, maxlen=seq_length, padding='pre') y_pred = np.argmax(model.predict(encoded), axis=-1) predicted_word = "" for word, index in token.word_index.items(): if index == y_pred: predicted_word = word break seed_text = seed_text + ' ' + predicted_word text.append(predicted_word) seed_text = text[-1] text = ' '.join(text) print(text)
seed_text = 'i love you' generate_poetry(seed_text, 5)
is no and i want to do is wash your name i set fire to the beat tears are gonna understand last night she let the sky fall when it was just like a song i was so scared to make us grow from the arms of your love to
Watch Full Course Here: http://bitly.com/nlp_intro
2 Comments