Use of Linear and Logistic Regression Coefficients with Lasso (L1) and Ridge (L2) Regularization for Feature Selection in Machine Learning
Watch Full Playlist: https://www.youtube.com/playlist?list=PLc2rvfiptPSQYzmDIFuq2PqN2n28ZjxDH
Linear Regression
Let's first understand what exactly linear regression
is, it is a straight forward approach to predict the response y
on the basis of different prediction variables such x
and ε. . There is a linear relation between x and y.
𝑦𝑖 = 𝛽0 + 𝛽1.𝑋𝑖 + 𝜀𝑖
y = dependent variable
β0 = population of intercept
βi = population of co-efficient
x = independent variable
εi= Random error
Basic Assumptions
- Linear relationship with the target y
- Feature space X should have gaussian distribution
- Features are not correlated with other
- Features are in same scale i.e. have same variance
Lasso (L1) and Ridge (L2) Regularization
Regularization is a technique to discourage the complexity
of the model. It does this by penalizing the loss function
. This helps to solve the overfitting
problem.
- L1 regularization (also called Lasso)
Itshrinks
the co-efficients which are less important tozero
. That means withLasso regularization
we canremove
some features. - L2 regularization (also called Ridge)
It does'treduce
the co-efficients tozero
but it reduces theregression co-efficients
with this reduction we can identofy which feature has more important. - L1/L2 regularization (also called Elastic net)
A regression model that uses L1 regularization technique is called Lasso Regression
and model which uses L2 is called Ridge Regression
.
What is Lasso Regularisation
3 sources of error
- Noise:
We can't do anything with the noise. Let's focus on following errors. - Bias error:
It is useful to quantify how much on anaverage
are the predicted values different from the actual value. - Variance:
On the other side quantifies how are the prediction made on thesame observation
different from each other.
Now we will try to understand bias - variance
trade off from the following figure.
By increasing model complexity
, total error will decrease
till some point and then it will start to increase
. W need to select optimum model complexity
to get less error.
If you are getting high bias then you have a fair chance to increase model complexity
. And otherside it you are getting high variance
, you need to decrease model complexity
that's how any machine learning algorithm works.
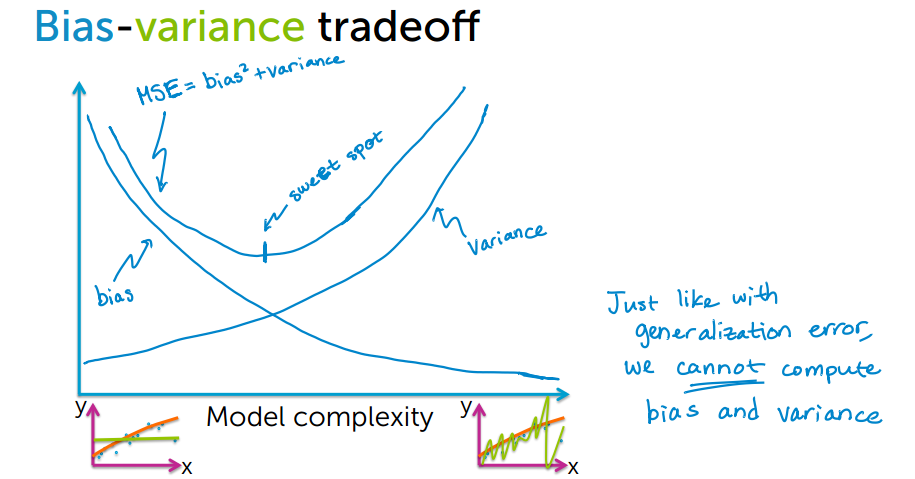

The L1 regularization adds a penalty equal to the sum
of the absolute value of the coefficients
.
We can observe from the following figure. The L1 regularization will shrink
some parameters to zero
. Hence some variables will not play any role in the model to get final output, L1 regression
can be seen as a way to select features in a model.
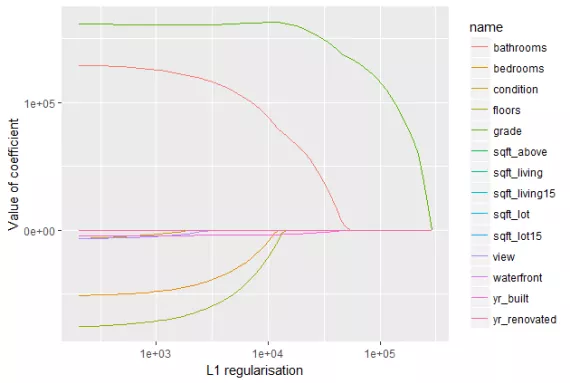
Let's observe the evolution of test error by changing the value of λ from the following figure.
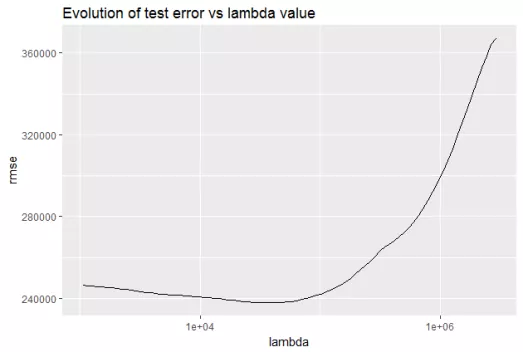
How to choose λ
Let's move ahead and choose the best λ.
We have a sufficient amount of data. In that we can split our data into 3
sets those are
- Training set
- Validation set
- Test set
- In the training set, we fit our model and set
regression co-efficients
with theregularization
. - Then we test our model's
performance
to select λ
- on
validation set
, if any thing wrong with the model likeless accuracy
we validate on thevalidation set
then we change the parameter the we go back to thetraining set
and so the optimization. - Finally, it will do generalize testing on the
test set
.
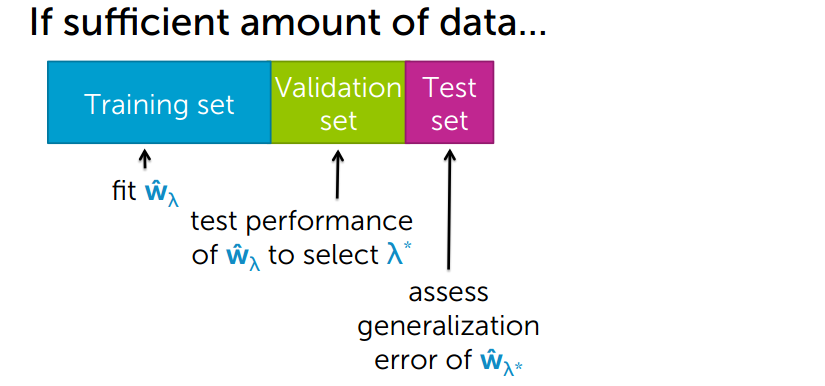
What is Ridge Regularisation
Let's first understand what exactly Ridge regularization
:
The L2 regularization
adds a penalty equal to the sum of the squared value of the coefficients
.
λ is the tuning parameter
or optimization parameter
.
w is the regression co-efficient
.
In this regularization,
if λ is high
then we will get high
bias and low
variance.
if λ is low
then we will get low
bias and high
variance
So what we do we will find out the optimized value of λ by tuning the parameters. And we can say λ is the strength of the regularization
.
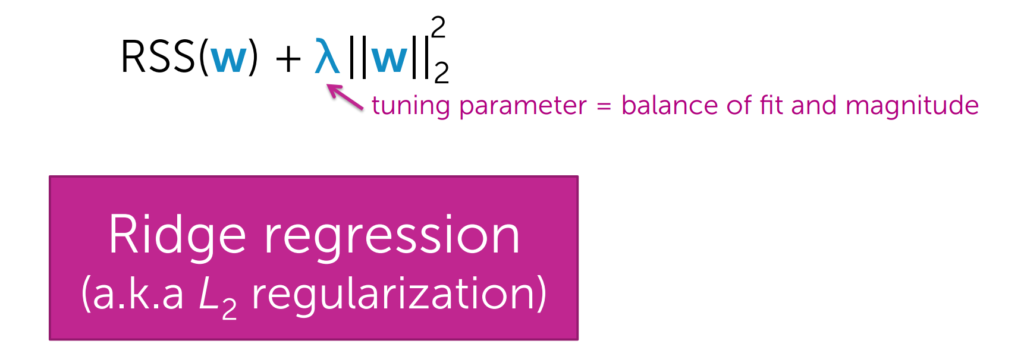
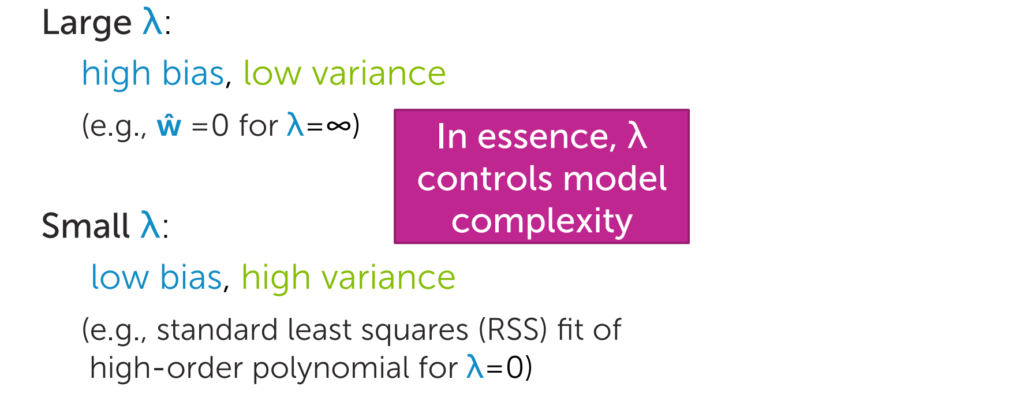
The L2 regularization will force the parameters to be relatively small
, the bigger the penalization, the smaller (and the more robust) the coefficients are.
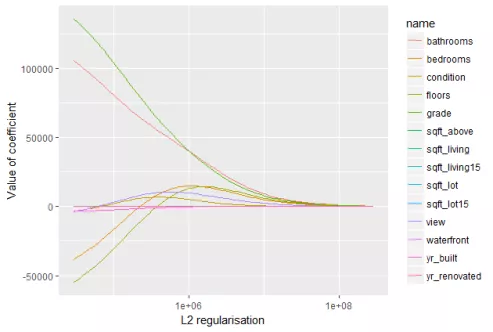
Difference between L1 and L2 regularization
Let's discuss the difference between L1 and L2 regularization:
L1 Regularization | L2 Regularization |
---|---|
It penalizes sum of absolute value of weights | It regularization penalizes sum of square weights |
It has a sparse solution | It has a non sparse solution |
It has multiple solutions | It has one solution |
It has built in feature selection | It has no feature selection |
It is robust to outliers | It is not robust to outliers |
It generates model that are simple and interpretable but cannot learn complex patterns | It gives better prediction when output variable is a function of all input features |
Load the dataset
Loading required libraries
import numpy as np import pandas as pd import seaborn as sns import matplotlib.pyplot as plt %matplotlib inline
from sklearn.model_selection import train_test_split from sklearn.ensemble import RandomForestClassifier from sklearn.feature_selection import SelectKBest, SelectPercentile from sklearn.metrics import accuracy_score
from sklearn.linear_model import LinearRegression, LogisticRegression from sklearn.feature_selection import SelectFromModel
titanic = sns.load_dataset('titanic') titanic.head()
survived | pclass | sex | age | sibsp | parch | fare | embarked | class | who | adult_male | deck | embark_town | alive | alone | |
---|---|---|---|---|---|---|---|---|---|---|---|---|---|---|---|
0 | 0 | 3 | male | 22.0 | 1 | 0 | 7.2500 | S | Third | man | True | NaN | Southampton | no | False |
1 | 1 | 1 | female | 38.0 | 1 | 0 | 71.2833 | C | First | woman | False | C | Cherbourg | yes | False |
2 | 1 | 3 | female | 26.0 | 0 | 0 | 7.9250 | S | Third | woman | False | NaN | Southampton | yes | True |
3 | 1 | 1 | female | 35.0 | 1 | 0 | 53.1000 | S | First | woman | False | C | Southampton | yes | False |
4 | 0 | 3 | male | 35.0 | 0 | 0 | 8.0500 | S | Third | man | True | NaN | Southampton | no | True |
titanic.isnull().sum()
survived 0 pclass 0 sex 0 age 177 sibsp 0 parch 0 fare 0 embarked 2 class 0 who 0 adult_male 0 deck 688 embark_town 2 alive 0 alone 0 dtype: int64
Let's remove age and deck from the feature list
titanic.drop(labels = ['age', 'deck'], axis = 1, inplace = True) titanic = titanic.dropna() titanic.isnull().sum()
survived 0 pclass 0 sex 0 sibsp 0 parch 0 fare 0 embarked 0 class 0 who 0 adult_male 0 embark_town 0 alive 0 alone 0 dtype: int64
Let's get the features of the data
data = titanic[['pclass', 'sex', 'sibsp', 'parch', 'embarked', 'who', 'alone']].copy() data.head()
pclass | sex | sibsp | parch | embarked | who | alone | |
---|---|---|---|---|---|---|---|
0 | 3 | male | 1 | 0 | S | man | False |
1 | 1 | female | 1 | 0 | C | woman | False |
2 | 3 | female | 0 | 0 | S | woman | True |
3 | 1 | female | 1 | 0 | S | woman | False |
4 | 3 | male | 0 | 0 | S | man | True |
data.isnull().sum()
pclass 0 sex 0 sibsp 0 parch 0 embarked 0 who 0 alone 0 dtype: int64
sex = {'male': 0, 'female': 1} data['sex'] = data['sex'].map(sex) data.head()
pclass | sex | sibsp | parch | embarked | who | alone | |
---|---|---|---|---|---|---|---|
0 | 3 | 0 | 1 | 0 | S | man | False |
1 | 1 | 1 | 1 | 0 | C | woman | False |
2 | 3 | 1 | 0 | 0 | S | woman | True |
3 | 1 | 1 | 1 | 0 | S | woman | False |
4 | 3 | 0 | 0 | 0 | S | man | True |
ports = {'S': 0, 'C': 1, 'Q': 2} data['embarked'] = data['embarked'].map(ports) who = {'man': 0, 'woman': 1, 'child': 2} data['who'] = data['who'].map(who) alone = {True: 1, False: 0} data['alone'] = data['alone'].map(alone) data.head()
pclass | sex | sibsp | parch | embarked | who | alone | |
---|---|---|---|---|---|---|---|
0 | 3 | 0 | 1 | 0 | 0 | 0 | 0 |
1 | 1 | 1 | 1 | 0 | 1 | 1 | 0 |
2 | 3 | 1 | 0 | 0 | 0 | 1 | 1 |
3 | 1 | 1 | 1 | 0 | 0 | 1 | 0 |
4 | 3 | 0 | 0 | 0 | 0 | 0 | 1 |
Let's read the data into x
X = data.copy() y = titanic['survived'] X.shape, y.shape
((889, 7), (889,))
X_train, X_test, y_train, y_test = train_test_split(X, y, test_size = 0.3, random_state = 43)
Estimation of coefficients of Linear Regression
First we will go with estimation of coefficients of linear regression
sel = SelectFromModel(LinearRegression())
Let's go ahead and fit the model
sel.fit(X_train, y_train) SelectFromModel(estimator=LinearRegression(copy_X=True, fit_intercept=True, n_jobs=None, normalize=False), max_features=None, norm_order=1, prefit=False, threshold=None)
With these we have trained our model
Let's see the features of the model
sel.get_support()
array([ True, True, False, False, False, True, False])
Now we will try to get the co-efficients
sel.estimator_.coef_
array([-0.13750402, 0.26606466, -0.07470416, -0.0668525 , 0.04793674, 0.23857799, -0.12929595])
Let's get the mean of the co-efficients
mean = np.mean(np.abs(sel.estimator_.coef_)) mean
0.13727657291370804
And calculate the absolute value of the co-efficients
np.abs(sel.estimator_.coef_)
array([0.13750402, 0.26606466, 0.07470416, 0.0668525 , 0.04793674, 0.23857799, 0.12929595])
features = X_train.columns[sel.get_support()] features
Index(['pclass', 'sex', 'who'], dtype='object')
Let's get the transformed version of x_train and x_test.
X_train_reg = sel.transform(X_train) X_test_reg = sel.transform(X_test) X_test_reg.shape
(267, 3)
Let's implement the randomForest
function and we wil do the training of the model.
def run_randomForest(X_train, X_test, y_train, y_test): clf = RandomForestClassifier(n_estimators=100, random_state=0, n_jobs=-1) clf = clf.fit(X_train, y_train) y_pred = clf.predict(X_test) print('Accuracy: ', accuracy_score(y_test, y_pred))
%%time run_randomForest(X_train_reg, X_test_reg, y_train, y_test)
Accuracy: 0.8239700374531835 Wall time: 250 ms
Now we will get the accuracy and wall time on the original data set
%%time run_randomForest(X_train, X_test, y_train, y_test)
Accuracy: 0.8239700374531835 Wall time: 252 ms
X_train.shape
(622, 7)
Logistic Regression Coefficient with L1 Regularization
Let's move ahead with the L1 regularization to select the features.
sel = SelectFromModel(LogisticRegression(penalty = 'l1', C = 0.05, solver = 'liblinear')) sel.fit(X_train, y_train) sel.get_support()
array([ True, True, True, False, False, True, False])
Let's get the regression co-efficients.
sel.estimator_.coef_
array([[-0.54045394, 0.78039608, -0.14081954, 0., 0. ,0.94106713, 0.]])
Let's get the transformed version of x_train and x_test by using the function transform()
X_train_l1 = sel.transform(X_train) X_test_l1 = sel.transform(X_test)
Now we will get the accuracy
%%time run_randomForest(X_train_l1, X_test_l1, y_train, y_test)
Accuracy: 0.8277153558052435 Wall time: 251 ms
L2 Regularization
Let's move ahead with the L2 regularization to select the features.
sel = SelectFromModel(LogisticRegression(penalty = 'l2', C = 0.05, solver = 'liblinear')) sel.fit(X_train, y_train) sel.get_support()
array([ True, True, False, False, False, True, False])
sel.estimator_.coef_
array([[-0.55749685, 0.85692344, -0.30436065, -0.11841967, 0.2435823 , 1.00124155, -0.29875898]])
X_train_l1 = sel.transform(X_train) X_test_l1 = sel.transform(X_test)
Let's check the accuracy of the data
%%time run_randomForest(X_train_l1, X_test_l1, y_train, y_test)
Accuracy: 0.8239700374531835 Wall time: 250 ms
1 Comment